Article: The Environmental Impact of AI and How to Minimize It: A Comprehensive Guide
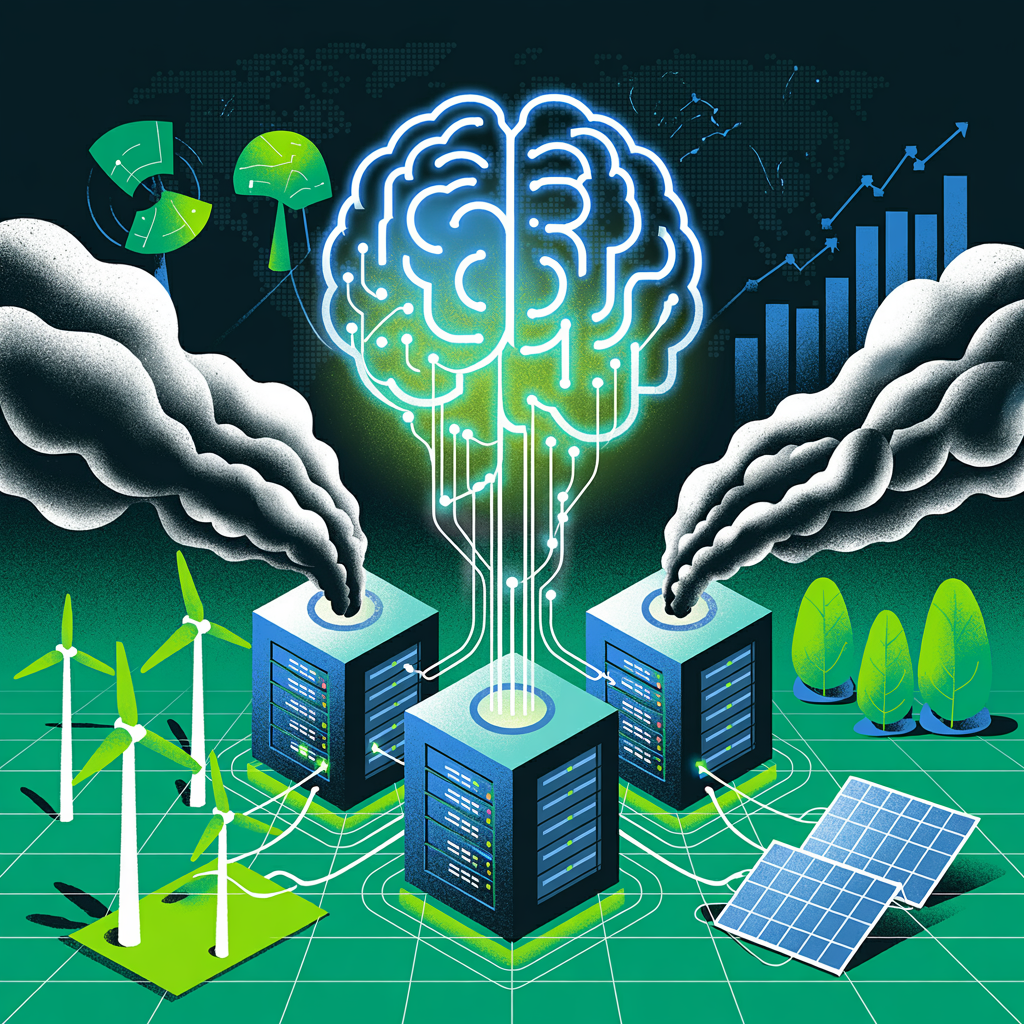
The Environmental Impact of AI and How to Minimize It: A Comprehensive Guide
As artificial intelligence transforms our world with remarkable innovations, it simultaneously raises concerns about its environmental footprint. While AI powers breakthroughs across industries, its energy-intensive nature creates a paradox: the very technology that could help solve climate challenges may be exacerbating them. This blog explores the substantial environmental impacts of AI systems and provides actionable strategies to minimize these effects while continuing to harness AI's transformative potential.
The Carbon Footprint of Modern AI
The environmental cost of artificial intelligence has reached alarming levels, with energy consumption and carbon emissions becoming increasingly significant as AI deployment expands globally.
Scale of Emissions
Training a large AI model produces approximately 283 tons of carbon dioxide—equivalent to 300 round-trip flights between New York and San Francisco, or nearly 5 times the lifetime emissions of the average car1. Recent studies have highlighted how specific models contribute to this footprint. For instance, BERT, a language model trained in 2019, required energy comparable to "a round-trip transcontinental flight"1. More concerning is that the computational requirements for advanced AI models double approximately every 3.4 months, leading to exponential growth in power usage and resulting carbon footprint1.
Popular AI Services' Environmental Impact
ChatGPT's environmental impact provides a startling case study of AI's carbon cost. This popular service generates over 260,930 kilograms of CO₂ monthly—equivalent to 260 flights between New York and London3. While each individual interaction seems minimal (ChatGPT produces just 1.59 grams of CO₂ per page view), the platform's massive user base of 164 million monthly users creates a substantial cumulative environmental impact3. Other AI platforms show even higher per-interaction emissions: Rytr generates 10.1 grams of CO₂ per page view, while Spellbook produces 6.5 grams—significantly higher than ChatGPT's per-view impact3.
The Growing Energy Demand
Behind AI's carbon emissions lies an increasingly energy-hungry data center infrastructure. According to the 2024 United States Data Centre Energy Usage Report, data center energy consumption more than doubled between 2017 and 20233. By 2027, energy costs for AI could increase to 85–134 Twh, nearly 0.5% of all current electricity usage1. The International Energy Agency predicts AI technologies, alongside cryptocurrency operations, could double energy consumption by 2026, with data centers accounting for one-third of this increase3.
Understanding AI's Energy Consumption Lifecycle
To effectively address AI's environmental impact, we must understand where and how energy is consumed throughout an AI system's lifecycle.
Training vs. Inference
AI's energy use can be separated into two main phases: training and inference. During training, AI models learn from vast datasets over periods that can last months depending on data complexity and volume2. Once trained, a model consumes energy each time it generates a new response or "inference"2. While training is extremely energy-intensive, the World Economic Forum estimates it comprises only about 20% of an AI model's overall energy use across its lifespan, with inferencing making up the remaining 80%2. A ChatGPT inquiry reportedly requires up to 10 times the electricity of a Google search to respond to a typical request2.
Factors Affecting Environmental Impact
Several factors influence an AI system's overall environmental footprint:
-
Model Size and Complexity: Larger models with more parameters require significantly more computational resources and energy2.
-
Geographic Location: AI models running in regions predominantly using fossil fuels have a much higher carbon footprint than those operating in places with cleaner energy sources1.
-
Query Volume: A model seeing more traffic generally requires more energy than a less active one2.
-
Hardware Efficiency: The number of processors supporting the model, along with their speed and power draw, influence the energy needed for each inference2.
Strategies for Minimizing AI's Environmental Impact
Fortunately, there are numerous approaches to reduce AI's environmental footprint while maintaining its benefits—a concept known as "Green AI" or "Sustainable AI."
Efficient Model Design and Development
One of the most effective strategies for reducing AI's environmental impact begins at the design phase:
-
Model Optimization: Building smaller, more efficient AI models that require less computational resources can dramatically reduce energy requirements without sacrificing performance2. Techniques like model compression and quantization significantly lower energy consumption by reducing the size of AI models and decreasing the computational resources needed to process them7.
-
Foundational Models vs. Custom Training: Opting to adapt existing foundational models rather than training new ones from scratch avoids much of the energy needed for development and spreads the energy use across the model's life2.
-
Retrieval-Augmented Generation (RAG): This energy-saving training method connects an AI neural network to new knowledge bases without requiring full retraining2.
-
Dimensional Reduction: Transforming data from high-dimensional space to low-dimensional space streamlines processing and improves efficiency2.
-
Batch Processing: Where appropriate, designing AI applications for batch processing instead of more energy-intensive real-time processing can reduce overall energy consumption2.
Infrastructure and Operational Considerations
Beyond model design, the infrastructure supporting AI systems plays a crucial role in their environmental impact:
-
Energy-Efficient Architectures: A model's platform architecture determines how efficiently it will use underlying hardware resources. Choosing energy-efficient architectures can significantly reduce environmental impact and operational costs2.
-
Sustainable Data Centers: Moving AI operations to energy-efficient data centers can substantially reduce carbon emissions. Data centers in regions with access to renewable energy sources (like hydroelectricity in Montreal, Canada) offer significant environmental advantages7.
-
Renewable Energy Integration: Using clean energy sources like wind, solar, or hydroelectric power to run AI infrastructure dramatically reduces its carbon footprint7.
-
Longevity-Focused Design: Creating models designed for long-term use reduces the need for frequent retraining and redeployment, lowering the overall environmental impact throughout the AI lifecycle2.
Balancing Performance and Sustainability
Finding the right balance between AI capability and environmental impact is crucial:
-
Right-Sizing Models: Determining the minimum model size and complexity needed to achieve desired outcomes prevents unnecessary resource consumption2.
-
Accuracy-Efficiency Tradeoffs: AI models may be modified for less environmental impact at the cost of some accuracy. Finding the optimal balance between performance and environmental impact is a key consideration for sustainable AI development1.
-
Lifecycle Assessment: Considering the full environmental impact of AI systems throughout their lifecycle—from development through deployment and ongoing use—can help identify opportunities for improvement5.
The Business Case for Green AI
Implementing sustainable AI practices isn't just environmentally responsible—it also makes business sense:
-
Energy Cost Reduction: As AI usage grows, the energy costs associated with running AI systems will increase substantially. Energy-efficient AI design can insulate businesses from these rising costs2.
-
Operational Efficiency: More efficient AI systems often run faster and more reliably, improving overall performance while reducing environmental impact4.
-
Regulatory Preparation: As environmental regulations tighten globally, organizations with sustainable AI practices will be better positioned to meet compliance requirements5.
-
Competitive Differentiation: With more than 76% of IT decision makers considering AI "critical" or "very important" for their organization's sustainable transformation, companies demonstrating AI sustainability leadership may gain competitive advantages6.
Conclusion: The Path Forward
The environmental impact of AI presents both challenges and opportunities. While current trends in AI development and deployment raise serious environmental concerns, the emerging field of Green AI offers promising pathways toward more sustainable artificial intelligence.
By adopting energy-efficient architectures, optimizing models, leveraging renewable energy, and carefully balancing performance with sustainability, we can minimize AI's environmental footprint while maximizing its benefits. The key lies in integrating sustainability considerations throughout the AI lifecycle—from initial design through ongoing operation.
As AI continues to transform our world, ensuring this transformation occurs in an environmentally responsible manner isn't just an ethical imperative—it's essential for the long-term viability of AI itself. By embracing sustainable approaches to AI development and deployment now, we can help ensure that artificial intelligence becomes a powerful ally in building a more sustainable future rather than an additional burden on our planet's already strained resources.
Citations:
- https://en.wikipedia.org/wiki/Environmental_impact_of_artificial_intelligence
- https://www.informationweek.com/sustainability/reducing-the-environmental-impact-of-artificial-intelligence
- https://sustainability-news.net/climate-nature/chatgpts-monthly-carbon-footprint-equivalent-to-260-transatlantic-flights/
- https://carboncredits.com/green-ai-explained-fueling-innovation-with-a-smaller-carbon-footprint/
- https://kpmg.com/nl/en/home/insights/2023/10/decoding-sustainable-ai-vs-ai-for-sustainability.html
- https://blogs.idc.com/2024/04/24/sustainable-ai-and-ai-for-sustainability/
- https://blog.premai.io/ai-sustainability-reducing-carbon-footprint-and-driving-innovation/
- https://time.com/7201501/ai-buildings-energy-efficiency/
- https://webosmotic.com/blog/sustainable-computing/
- https://deepgram.com/ai-glossary/eco-friendly-ai
- https://news.mit.edu/2025/explained-generative-ai-environmental-impact-0117
- https://www.iec.ch/blog/importance-sustainable-ai
- https://www.unep.org/news-and-stories/story/ai-has-environmental-problem-heres-what-world-can-do-about
- https://corporate-blog.global.fujitsu.com/fgb/2024-12-18/01/
- https://earth.org/the-green-dilemma-can-ai-fulfil-its-potential-without-harming-the-environment/
- https://blogs.microsoft.com/on-the-issues/2023/11/16/accelerating-sustainability-ai-playbook/
- https://hbr.org/2024/07/the-uneven-distribution-of-ais-environmental-impacts
- https://www.scientificamerican.com/article/ais-climate-impact-goes-beyond-its-emissions/
- https://corporate.britishcouncil.org/insights/minimising-ai-bias-best-practices-organisations
- https://www.bbc.com/news/articles/c51yvz51k2xo
- https://www.forbes.com/sites/elenabou/2024/12/16/green-ai-the-yin-yang-of-a-breakthrough/
- https://accesspartnership.com/12-key-principles-for-sustainable-ai/
- https://www.ey.com/en_nl/insights/climate-change-sustainability-services/ai-and-sustainability-opportunities-challenges-and-impact
- https://scet.berkeley.edu/reducing-ais-climate-impact-everything-you-always-wanted-to-know-but-were-afraid-to-ask/
- https://www.nature.com/articles/s41598-024-54271-x
- https://greenai.cloud
- https://www.techtarget.com/searchenterpriseai/definition/sustainable-AI
- https://environment.yale.edu/news/article/can-we-mitigate-ais-environmental-impacts
- https://www.datacamp.com/blog/sustainable-ai
- https://transformainsights.com/blog/ai-sustainability-challenges-opportunities
- https://www.statworx.com/en/content-hub/blog/how-to-reduce-the-ai-carbon-footprint-as-a-data-scientist
- https://www.weforum.org/stories/2024/07/generative-ai-energy-emissions/
- https://www.phoenix-ai.com/green-computing
- https://pecb.com/article/eco-innovation-how-ai-is-shaping-sustainable-practices
- https://lamarr-institute.org/blog-tag/sustainable-ai/
- https://www.sustamize.com/blog/6-ways-ai-can-help-reduce-carbon-emissions
- https://www.group.sener/en/insights/how-can-artificial-intelligence-help-us-to-improve-energy-efficiency/
- https://metalab.essec.edu/green-ai-computing-where-are-the-pathways/
- https://webosmotic.com/blog/eco-friendly-ai-sustainability/
- https://stayrelevant.globant.com/en/technology/sustainable-business/sustainable-ai-for-a-sustainable-future/